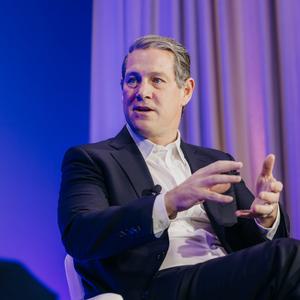
Generate:Biomedicines
We could generate novel protein therapeutics using new computational tools, without having to discover them through trial and error?
Machine-learning algorithms trained on all known protein sequences and structures can generate novel DNA sequences for proteins never seen in nature, providing the precise therapeutic solution for any problem.
Life works in three dimensions. Say you want to create a drug that interrupts a specific biological process, such as the way the virus SARS-Cov-2, which causes COVID-19, uses its spike proteins to latch onto human ACE2 receptors and break into cells. Perhaps your specific goal is to disrupt the virus by hitting it with a molecule that will stick to the spike proteins—like a Velcro fabric strip attaching to its hooks—and prevent them from binding to ACE2.
To develop such a molecule, you would need to understand the 3D structure of the spike proteins, and how exactly they make contact with ACE2. Then you would have to find—or design—a new protein with a customized shape that fits tightly into the major surface features (called epitopes) on the spikes.
The catch is that our sense of the way cells store the specifications for proteins is mostly one-dimensional. We know that the four-nucleotide genetic code in DNA is transcribed into RNA, and that triplets of RNA are translated into 20 standard amino acids, which then form chains that can fold up into millions of potential proteins. What we don’t understand are the convoluted rules governing the way a specific chain of amino acids assumes the three-dimensional shape that will allow it to do its job as a particular protein.
It would be nice if we could predict the function of a protein-based drug solely from its amino-acid sequence, or synthesize a chain of amino acids that would fold up into the exact shape we want—but those are both hard problems. The physics and geometry involved are nearly intractable. “A holy grail in biology has been what people have called the protein folding challenge,” says Geoffrey von Maltzahn, a general partner at ĢƵAPP. “How does a DNA sequence encode the underlying three-dimensional structure of a protein? Even harder: What’s its function in a biological system?”
That challenge might take decades to solve using traditional methods in biophysics. Fortunately, there is now an alternative. Machine learning, a domain of artificial intelligence that employs methodologies from data science to empower computers to recognize patterns and generate complex new things, liberates scientists and engineers so that they can leapfrog over the details of the sequence-structure-function problem.
Machine-learning algorithms running on powerful processors can analyze hundreds of millions of known proteins, looking for statistical patterns linking sequence, structure, and function. In much the same way that the patterns found in large libraries of songs, texts, or photographs have been used to create AI-generated music, language, and faces, a new ĢƵAPP company, Generate:Biomedicines, is showing that patterns in protein sequences can be used as springboards for the design of custom protein drugs of any variety—from short peptides to complex antibodies, enzymes, and cytokines.
This, in turn, offers the potential to rationally create and test entirely new medicines that exactly meet therapeutic needs. Traditional protein drug discovery methods rely on trial-and-error processes such as high-throughput screening or manipulating the immune systems of transgenic animals.
Generate:Biomedicines has proved that its machine-learning platform can generate new biological molecules with therapeutic value, says von Maltzahn, who is Generate:Biomedicines’ co-founder and co-CEO. “We want people to be able to say, ‘All right. Wow. You can simultaneously predict antibodies, peptides, or other binders that hit 10 different sites on a target protein. That has never been possible before and will lead to much more precise and potent therapeutics.’” The company has already demonstrated it can generate antibodies and peptides against a dozen targets, offering better alternatives to existing therapeutics as well as drugging targets that were impervious to traditional discovery methods. Next, von Maltzahn says, the company will invest in developing and testing its own drugs and taking them to market, while also exploring opportunities to partner with other drug makers.
Generate:Biomedicines is itself a chimera: the product of two exploratory projects within Flagship that in 2019 fused into a single company. This hybrid heritage helps to explain the startup’s distinctive approach to what’s being called “generative biology.”
One project, originally code-named FL56, was led by another Flagship general partner, Avak Kahvejian, and built around the insights of Gevorg Grigoryan, a biochemist and computer scientist at Dartmouth College. Back in 2016, after an enormous statistical effort to analyze all of the molecules in the Protein Data Bank, a global repository of 3D structural information, Grigoryan and his colleagues at Dartmouth discovered that protein structure forms according to a kind of language. “We found that natural folded proteins reuse the same design elements over and over—tertiary structural motifs,” Grigoryan says. Incredibly, the discovery of this language enabled the researchers to engineer novel proteins that fold and function entirely without resorting to any physical descriptions. “This mean that for the first time, we could make sophisticated inferences about the relationship between sequence and structure without needing to understand it in an atomistic way,” Grigoryan says.
“Imagine a periodic table of elements of protein structure, if you will,” Kahvejian adds. “We could take any of those elements, put them together, create a certain protein, and reshuffle those elements and create another protein. In this way we could describe about 50 to 60 percent of all proteins in the world.”
The idea behind FL56 was to use Grigoryan’s findings as the basis for an algorithmic drug discovery platform. Kahvejian says, “If you knew one protein, could you find new motifs that would interact with that protein? We started working very closely with Grigoryan on protein-protein interaction prediction, and asked whether this could be directly applied to the creation of antibodies to any target, at will.”
At the same time, Molly Gibson, a principal at Flagship, was working with von Maltzahn on a different project to investigate whether machine learning could help biologists get beyond the limitations of the more traditional approaches to protein structure prediction. Since the late 1990s, researchers have been using software such as Rosetta to model simulated proteins based solely on an understanding of the atomic-level forces between amino acids. “It turns out that’s really challenging for a lot of reasons,” Gibson says. “One is because there’s a lot of physics in the way proteins behave that we’re still learning. Also, the computer power that’s needed to simulate those interactions as you get to larger and larger proteins becomes really limiting. So you start to make approximations, and as those approximations propagate through a larger protein, you can get larger and larger errors.”
Gibson and von Maltzahn’s project, code-named FL57, was designed to test whether the enormous strides being made in machine learning in areas like natural language processing and image processing could be applied to the amino-acid sequences of proteins. Their early proof-of-concept experiments “just took thousands and thousands of protein sequences and learned directly from those to try to predict the function—not telling you anything about the structure or its properties but still learning how to optimize them,” Gibson says.
At some point in 2018, the Flagship partners recognized that the two projects were organized around the same larger theme—the shift from a physics-based understanding of protein structure to a statistical one—and that, moreover, they had the same ultimate goal: building a generative platform for creating protein drugs.
“FL57 started with sequence, with the expectation of going to three-dimensional structure subsequently,” von Maltzahn says. “FL56 started with this Lego-block perspective on how structural motifs are composed in nature,” and it would have worked its way back to sequence predictions. “We realized that the two together would allow us to do something faster and bigger.”
So in 2019, FL56 and FL57 merged into a single company, with Kahvejian and von Maltzahn as co-founders and co-CEOs, Gibson as chief innovation officer, and Grigoryan as chief technology officer.
Today Generate:Biomedicines has over 30 employees divided into three groups: a machine learning team focused on developing computational models, a biological engineering team focused on generating more raw protein-structure data, and a medicines team focused on preclinical biology experiments. Kahvejian says the company expects that its statistical models will speed up the generation of candidate medicines in a range of therapeutic categories, including antibodies, peptides, enzymes, gene therapy, modular proteins, and cytokines. But he says the work is furthest along in the area of antibodies.
Which turns out to be timely. The company’s generative approach is exemplified by a project it undertook in February and March of 2020 to rapidly generate new antibodies to SARS-CoV-2—that is, molecules that could lock onto the spike proteins and impair the action of the coronavirus. “This was a perfect opportunity to demonstrate instantaneous generation of custom protein therapeutics toward an emerging target,” says Grigoryan.
The discovery part of the effort took just 17 days from beginning to end, according to Gibson. “The first three days was a combination of two things,” she says. “First was identifying what the target is and what we want to do—that we were going to go after the spike protein and we wanted to hit it on these epitopes. And then a really small portion of that time was the computational piece, which is almost instantaneous. In a matter of minutes, we generated on the order of 100 antibodies to two different locations on the spike protein.”
The process then slowed down a bit over the next 14 days, owing to the limitations of molecular biology and DNA synthesis. During that interval, Gibson explains, “we were actually building those candidate antibodies from the DNA, expressing them in cells, and then testing them in our assay systems” to see which ones bind most strongly to the SARS-CoV-2 receptor binding domain.
The process is much faster than the traditional high-throughput-screening approach to discovery. That’s because each of Generate:Biomedicines' 100 candidate molecules started out with a much greater chance of binding to the target—10 orders of magnitude greater than the hit rate for a typical discovery campaign.
Now the company is planning a full sequence of steps to take its generated SARS-CoV-2 antibodies to clinical testing. But that doesn’t mean Generate:Biomedicines will evolve into an antibody drug company.
“COVID antibodies are only one example of what a generative biology platform can do,” Kahvejian says. “We’ve wanted to avoid chasing shiny objects, and we don’t believe any product will ever be as valuable as a modality, nor any single modality as valuable as the underlying generative biology platform. But it’s a pandemic, and we wanted to do our part, considering the power of our platform and its direct applicability to the problem.”
In the future, the company will begin to sort through the forest of other applications of generative biology to find the most valuable trees. “We’ve proven beyond a reasonable doubt that our algorithms are applicable to novel antibodies, novel peptides, novel enzymes, and novel whole proteins, and to being able to create machinery in the gene editing realm,” von Maltzahn says.
Latest ĢƵAPP from Generate:Biomedicines
- Amgen and Generate Biomedicines Announce Multi-Target, Multi-Modality Research Collaboration Agreement 01.06.2022
- Generate Biomedicines Announces First External Equity Raise of $370 Million to Advance its Drug Generation Platform 11.18.2021
- ĢƵAPP Expands Leadership Team with Appointment of Michael T. Nally as CEO-Partner 03.31.2021
- ĢƵAPP’s Scientists Invent Machine Learning–Powered Platform to Generate Novel Biomedicines 09.09.2020